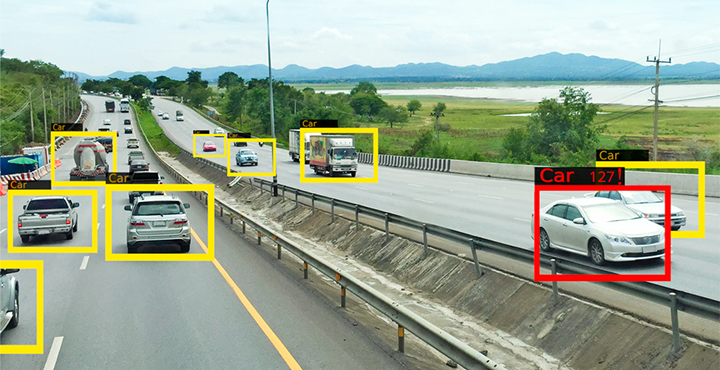
We are in the age of AI that is dependent on machine vision applications for camera systems. This technology surge has necessitated thinking about camera systems in new ways because machine vision that is based on neural networks has different requirements compared to human vision that is modeled for camera systems that generate images for perceptual interpretation.
Norman Koren, Imatest’s founder, has discovered novel applications of the relationship of image signal and noise in an image, particularly related to the metric of information capacity that is based on the Shannon-Hartley theorem. We define information capacity as the maximum amount of information that can pass through a channel (For imaging systems, this is the camera system.) without error. Simply put, it is a measure of a camera’s “goodness.” By measuring the signal and noise from the same slanted edge region of an image, more understanding can be gained for a given image regarding the fundamental capabilities of a camera system’s potential performance. While signal and noise measurements can be quantified independently, the traditional metrics of signal such as SFR and MTF50 are not indicative of the fundamental image information and, thus, diverge from the performance metrics of machine vision such as detection confidence levels.
At Imatest, we are working with partners in industry and academia to validate the ties between the family of image information metrics for a given camera system and the machine vision capabilities of the same system. These machine vision capabilities are based on the AI models that are trained for the system’s camera captures. One partner we want to highlight is the research group under Dr. Brian Deegan at the University of Galway, Ireland. Their lab is part of The Connaught Automotive Research (CAR). They have looked closely at the information capacity numbers for a variety of camera parameters and the metric correspondence to machine vision performance.
Figure 1 shows the simulation chart used in the Deegan et al. research, which contains sets of four different sizes of simulated people (used as the test objects) and a slanted edge used to make the information capacity measurements. A series of images with various degradation levels of contrast, Gaussian blur, and Poisson noise were generated and evaluated using a YoloV10m model. The confidence interval of object detection was obtained as a performance measurement of the model.
Fig. 1. A simulated test chart used for the Deegan et al. research that has four specific sets of differently sized people (Very large, Large, Medium, and Small) to quantify the machine vision performance and a slanted square with edges used to quantify the traditional image quality metrics and new image information metrics.
Figure 2 shows the relationship between machine vision performance and a traditional image quality metric, MTF50. For this plot, one can observe that the correlation of the model’s average confidence and MTF50 is low overall, which indicates the shortcoming of traditional metrics. Note that the data points with zero average confidence are the results for the small people category, which were too small to be detected by the machine vision model.
Fig. 2. Machine Vision confidence versus MTF50. For a test chart that has four specific sizes of people (Very large, Large, Medium, and Small), this graph shows that the machine vision model‘s confidence levels and MTF50 are not strongly correlated. Note: The data points with zero average confidence are the results for the small people category, which were too small to be detected by the machine vision model.
See Figure 3 for a similar comparison, but this time the machine vision model confidence is compared to the new Shannon image information capacity metric (SIC in the figure.). In this plot, the correlation of the machine vision model confidence and the new information capacity is high. The green data points are for the very large category of people size in the simulation chart. Similarly, the yellow data points of for the large category and the red data points are for the medium category. Again, the data points with zero average confidence (blue data points in this figure) are the results for the small people category, which were too small to be detected by the machine vision model. These sets of data indicate that information capacity can be used as a good metric for characterizing the machine vision that is part of AI.
Fig. 3. Machine Vision confidence versus Information Capacity. For a test chart that has four specific sizes of people (Very large, Large, Medium, and Small), this graph shows that the machine vision model‘s confidence levels correlate well with the Shannon information capacity (SIC) for each grouping of people size detection (with the exception of the detection of small people, which were too small to be detected by the machine vision model).
Imatest continues to work with our partners in academia and industry to further validate the image information metrics. In addition, we are leading the effort in the ISO/TC42 Photography standards body to bring these metrics into a new standard, ISO 23654 Image Information Metrics. Currently, this effort is in the new work initiative stage.
If you would like to collaborate with Imatest on the validation and standardization of image information metrics, reach out to us at infocap@imatest.com. You can also try out the image information metrics directly by using our Imatest analysis software that has the metrics included in our newer releases. See more details at imatest.com/imaging/image-information-metrics/.